QSP Life Use Cases
Providing quantum-based solutions to your most difficult challenges.
Jump to:
Challenge
Free Energy Perturbation (FEP), a computational technique with immense potential, has revolutionized drug discovery. By predicting relative binding energies for structurally similar ligands, FEP offers a powerful tool for understanding molecular interactions.
Despite its successes, FEP faces critical limitations. Molecular Mechanics (MM)-based FEP, while widely used, falls short in predictive accuracy for certain systems. Issues such as polarization, halogenation, π-stacking, and charge variations challenge classical MM force fields. To address these limitations, one can replace MM with QM in the binding region, using a hybrid QM/MM implementation. But there’s a catch: speed matters. Until now, no QM/MM hybrid has met the turnaround demands of modern drug discovery.
Solution
Our QUELO QM/MM-FEP platform has been developed to be a 1:1 replacement for inferior MM-FEP methods, using the same FEP approach, but replacing the MM representation of the ligand and binding site with quantum mechanics. In many cases where MM-FEP does not perform satisfactorily, QUELO is capable of providing much superior predictions. And it can do this at a modest increase in computational turnaround time.
FEP is based on the thermodynamic cycle shown in Figure 1. The horizontal processes corresponding to experimental observations for two similar ligands can be related to FEP calculations performed for the vertical processes because free energy is a state function. We have extensive experience applying our QUELO QM/MM-FEP platform to ligand binding for our customers, as well as for some validation work on publicly available data sets.
Results
In Figures 2-3, we compare the predictions using MM-FEP and QM/MM-FEP for CDK2, a well-studied ligand/protein system. Both correlation and Mean Unsigned Error (MUE) improve considerably when moving to QUELO. Both sets of results are obtained using the same starting coordinates, and standard FEP protocols.
In Figures 4-5. we compare the performance of MM-FEP and QM/MM-FEP for TNKS2, a ligand/protein system distinguished by the fact that the ligand set consists of molecules with differing formal charges. While MM struggles with “charge changes” such as those found in this set, QUELO provides good, useful, results, with impressive improvement in both correlation and mean unsigned error.
Figure 6 presents data obtained in an actual project carried out with a customer. This customer contacted QSimulate because they were not able to obtain satisfactory results for their system using a well-known MM-based FEP platform. The results using QUELO were far superior, and considerably more predictive, especially for the most tightly binding ligands. In fact, the correlation for the more tightly bound ligands is extremely good. The two outlier points are for some of the worst binders in the validation set, and the customer indicated that these data might reflect limitations of the assay in that range.
Challenge
The significance of covalent ligands in drug discovery continues to escalate, presenting a solution to the challenging targets that have eluded conventional non-covalent binding molecules. This subset of targets encompasses the notorious "undruggable" entities, characterized by either shallow or absent binding sites, or where the binding site architecture recurs across a broad spectrum of proteins (e.g., kinases), posing challenges for achieving specificity. Moreover, the burgeoning interest in covalent ligands extends to applications in molecular glues, PROTAC drugs, and interactions with nucleic acid targets.
Conventional molecular mechanics (MM) force fields inadequately address the intricate alterations in topology and electronic distribution inherent in covalent bond formation. For robust predictions concerning covalent ligand systems, reliance on quantum mechanics transcends being merely an alternative to MM—it stands as the singularly appropriate choice.
Solution
Clients seeking computational methodologies for characterizing covalent ligands frequently approach us subsequent to unsuccessful attempts at applying platforms rooted in classical methodologies.
Covalent binding is generally understood as being a two step process, with the first step being non-covalent orientation within the binding site, followed by the formation of a covalent bond. The covalent bond can be either reversible or irreversible, depending on the relative stabilities (energetics) of the unbound and bound forms. Figure 1 summarizes the energetic profile for irreversible covalent ligand binding.
Irreversible covalent ligand binders can be understood via the thermodynamic cycle shown in Figure 2. Of particular note is that because the rate constant for the reverse process of bond breaking after formation is effectively zero, the formation of the covalent bond is unidirectional, rather than reversible. To understand the bond formation process we must use QM tools to characterize the energetics associated with transition state (TS) formation. The rate constant for this process is termed “Kinact”.
A complex QM workflow is required to characterize the transition state. This workflow, which incorporates many steps and has until now been the province of a small number of QM experts in this type of work, has been automated in our platform.
Results
Experimental measurements for irreversible binding typically reflect a combination of both the non-covalent orientation step and the covalent bond formation, but in many cases, the energetics are dominated by the Kinact energetics, and prospectively useful correlation between the activation energy for the TS and the experimental readout is observed.
This can be seen in Figure 3. This reflects data that was calculated for a customer. The experimental data reflects whole-cell measurements and is expected, in the best case, to be casually correlated with the binding energetics. The customer was not able to obtain useful predictions using programs based on classical MM. With QuValent TS characterization, we obtained a correlation that the customer deemed impressive and useful was obtained.
Challenge
Covalent ligands are of increasing importance to drug discovery, offering the ability to address targets that have proven difficult or impossible to drug using molecules that bind non-covalently. This group of targets includes so-called “undruggable” targets–Shallow or no obvious binding site, or else, where the binding site geometry is repeated in a large class of proteins (e.g. kinases) and specificity is difficult. Increasingly, there is also interest in covalent ligands for molecular glues, PROTAC drugs, and to bind to nucleic acid targets.
Classical MM force fields do a poor job of dealing with the changes in topology and electronic distribution that accompany covalent bond formation. If one wants to make reliable predictions for covalent ligand systems, quantum mechanics is not just a good alternative to MM–it’s the only suitable choice.
Solution
Customers who need computational tools to characterize covalent ligands frequently come to us after trying without success to apply platforms based on classical methods.
Reversible covalent ligand binders can be understood via the thermodynamic cycle shown in Figure 1, which reduces to the simpler cycle in Figure 2. The simpler cycle is often sufficient if the focus is on predicting the overall changes in binding due to changes in the ligand.
Technically, one could try to apply MM methods to this simplified thermodynamic cycle. But if one does, you are likely to obtain little or no signal owing to the parameterization limitations of MM. To demonstrate this, we have applied both MM-FEP and our QuValent QM/MM-FEP to a recently published data set for reversibly covalent ligands (Fairhurst et al. (2020) J Med Chem. 63 12542). Simulations were run in a full periodic ligand + protein + explicit solvent model system.
Results
In the initial approach, we applied standard MM-FEP using the Amber ff14sb force field and GAFF2 parameters for the ligand. The results, as depicted in Figure 4, were disappointing—no discernible signal was observed.
When we switched to QuValent QM/MM-FEP for the same series, utilizing identical starting conformations and the same mutation map, the outcome was exceptional. Figure 5 showcases these impressive results.
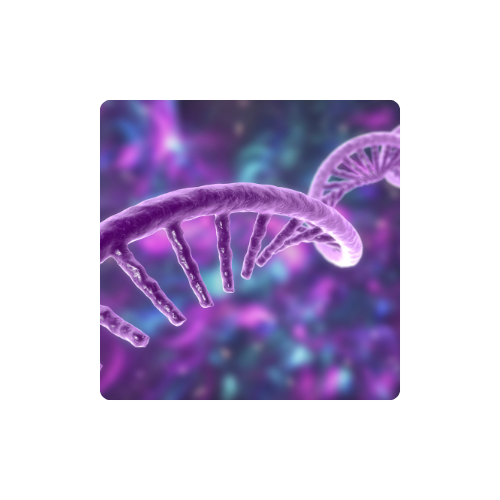
Challenge
In recent decades, RNA has emerged as a compelling alternative to proteins for drug discovery. While early efforts primarily centered around antisense RNA approaches, challenges related to delivery and stability have sparked interest in leveraging traditional small molecules for RNA targeting.
The unique properties of nucleic acids, including their highly polarized nature (including the charged PO4 group) and the significance of π-stacking interactions, pose challenges for classical computational methods.
Solution
Quantum mechanics, with its ability to account for electronic distribution, is better suited for modeling these intricate interactions. Moreover, quantum mechanics can handle covalently bound ligands—commonly used in RNA drug design.
The QSimulate platform accommodates both protein and nucleic acid targets (RNA or DNA). It seamlessly handles non-covalent and covalent ligands, even in complex systems like nucleic acid-protein complexes. To perform free energy calculations, you’ll need the target structure, a reference ligand bound to the target, and structures of other ligands of interest.
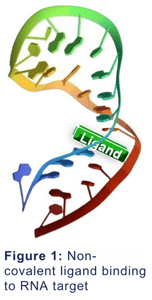
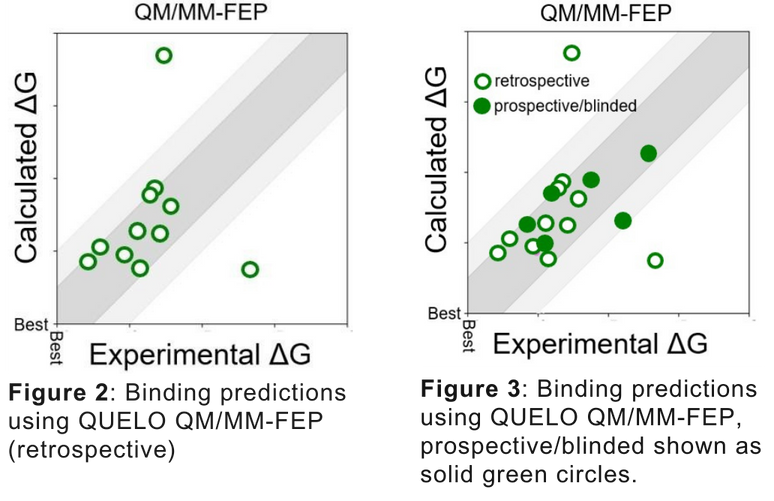
Results
QSimulate collaborates with customers on diverse nucleic acid targets. While specifics remain confidential, consider an example: non-covalent inhibitors designed to bind an RNA hairpin (see Figure 1).
Moving to the QUELO QM/MM approach improves agreement between experiment and prediction, as shown in Figure 2.
Finally, as a rigorous test of the QM/MM model, relative binding energies were predicted prospectively (blinded). These additional predictions are shown in Figure 3 as solid green circles. As can be seen, these predictions are equally as good as the retrospective validation.